INSIGHTS
Generative AI: The new transformation in business technology
In this series, ”Putting Generative AI to Work,” Altman Solon, the largest global TMT strategy consulting firm exclusively dedicated to the Telecommunications, Media, and Technology sectors, offers insight into how generative AI is revolutionizing business. Our team surveyed 292 senior business leaders and spoke to 21 industry experts to understand the adoption of generative AI tools for specific high-impact enterprise use cases. Altman Solon focused on four areas with strong momentum: software development, marketing, customer service, and product design, and found the highest adoption in software development and marketing and emerging adoption in customer service and product design.
Generative AI went mainstream in 2023. OpenAI’s ChatGPT made history in January by becoming the fastest-growing consumer application, ramping up to 100 million daily active users in just 40 days. Millions of people engaged in thought-provoking conversations, received jokes and poetry, and played games with a chatbot powered by OpenAI’s large language model. But aside from consumer delight, generative AI technology has already caught the business world’s attention and is being folded into company functions.
In March 2023, Altman Solon surveyed 292 senior executives and interviewed a panel of industry experts to better understand opportunities for generative AI in businesses. In this study, through analysing technological capabilities, and a long list of emerging use cases in functions as diverse as human resources, R&D, legal, and finance, Altman Solon investigated business activities with the most potential for immediate adoption and the highest momentum in the market: software development, marketing, customer service, and product management and design. These four functions rely on relatively more established generative AI solutions compared to other business activities. Our findings show that software development and marketing are the activities with the highest adoption rates of generative AI solutions. In the longer term, this technology will become increasingly suitable for customer service professionals and product designers and managers, who have lower generative AI adoption due to the perceived immaturity of available generative AI tools and satisfaction with existing tools.
Among organizations surveyed, there is a high intent to adopt AI tools in the future across all four business functions. While this is a positive indicator for enterprise generative AI, enthusiasm may not result in actual adoption due to resource constraints, internal barriers, or perceived risks.
The workings of Generative AI
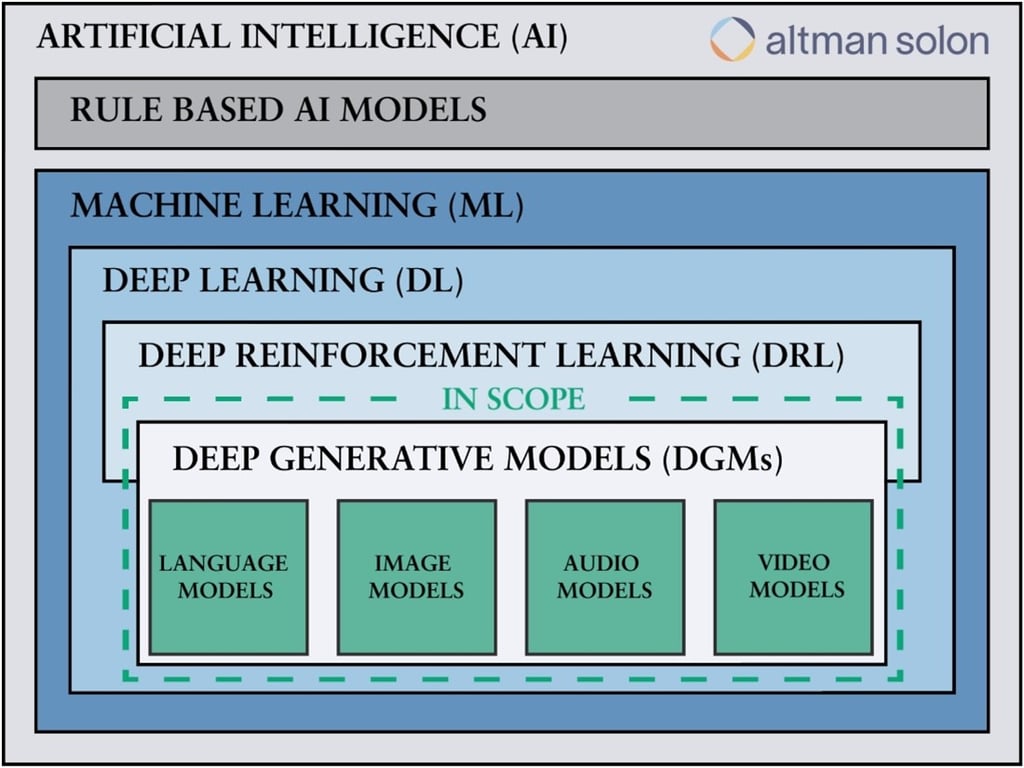
Generative AI is a subset of artificial intelligence that is distinguished by its ability to create new content based on a user’s prompt. While other AI models have been around longer, they are unable to create new content dynamically. For example, machine learning models have long been used to predict purchase likelihood, classify images into specific categories, discern the intent or sensitivity of a customer’s conversation with a call center operator, or provide pre-set responses based on pre-defined questions. These models all use data to ”learn” and improve their output, but none can generate new content. Generative AI’s content can uncannily imitate human ”chattiness” and preferences and produce quality visuals in response to user prompts.
Generative models can be categorized by the type of content they create, including (but not limited to) language, image, audio, video, or 3D. These five different model types can be characterized by their capabilities. For example, the core capabilities of language generation include retrieving responses through search, summarization, text translation (from one language to another, including code), and autocomplete functions. For image generation, users can convert text to images or alter existing images to produce a new image. Language and image capabilities are more mature today than other generative AI models like video or 3D shapes. We expect future generative AI models to be multimodal, combining various data types.
Over the past decade, enterprises have adopted artificial intelligence tools (not just generative AI) for improved productivity. Stanford University’s Artificial Intelligence Index Report 2023 findings show that enterprise adoption of AI tools has plateaued at 50% to 60%. However, due in part to generative AI’s novelty and ability to create content from prompts, it is a subset of AI expected to grow in enterprise adoption.
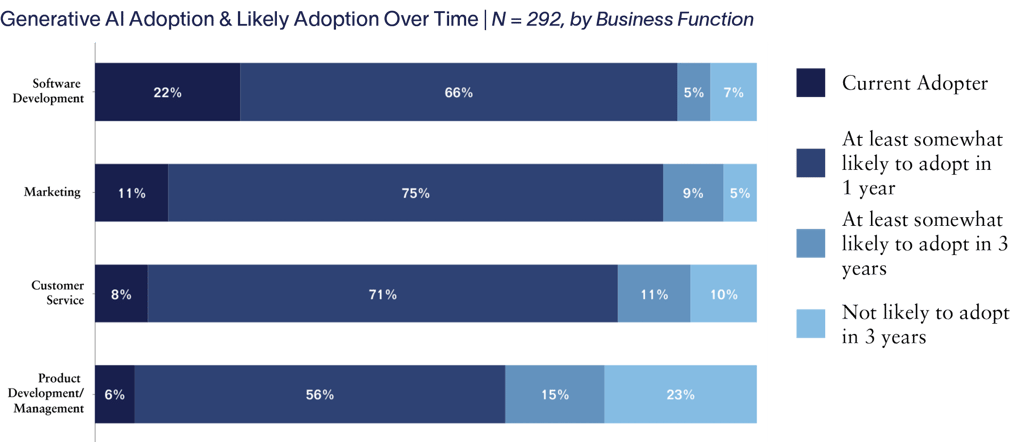
Generative AI can speed up code generation, creating value for software developers
Generative AI tools like GitHub Copilot or Amazon CodeWhisperer allow software developers to speed up production by automatically generating code from text input. With generative AI, some software code for front-end and back-end development can be completed or written from scratch using text prompts. In addition to generating new code, generative AI also can help automate code documentation, translation, testing, and debugging.
According to our research, generative AI in software development has the highest adoption, currently used by 22% of organizations surveyed. Software developers are accustomed to using coding tools to increase productivity compared to other business functions. In some cases, generative AI adds value to solutions already in use. For instance, when the coding database GitHub released its generative AI programming tool GitHub Copilot, it gained over 400,000 subscribers in the first month. 66% of surveyed organizations believe they are likely to adopt generative AI for development in the next year, and an additional 5% in the next three years. Currently, generative AI coding tools cannot replace human software engineers entirely, and they are unable to handle niche or proprietary languages. They are best suited to automating repetitive coding tasks.
”Generative AI code generation is ready for use. I’m seeing rapid adoption in both software engineering and enterprise software development. I believe it can improve productivity by two to threefold.”
— Senior Director of Software, Model Provider —
The most widely adopted use case within software development and all four examined business functions is code generation and completion, with current adoption at 16%. The next two most common use cases are code testing (9% current adoption) and code documentation (8% current adoption). Surveyed organizations singled out generative AI’s ability to increase speed in repetitive tasks and enhance engineers’ creativity by freeing up their time. 73% of software developers who have adopted or want to adopt generative AI cite increased speed. The rapid improvement in code quality produced by generative AI has convinced many that this use case will only grow in relevance. According to a Director of Product Management who has adopted generative AI, ”Two years ago, code-focused models performed like an average programmer. Today we’re seeing very high-quality output. We’re going to see the percentage of code written by machines grow significantly in the coming years.”
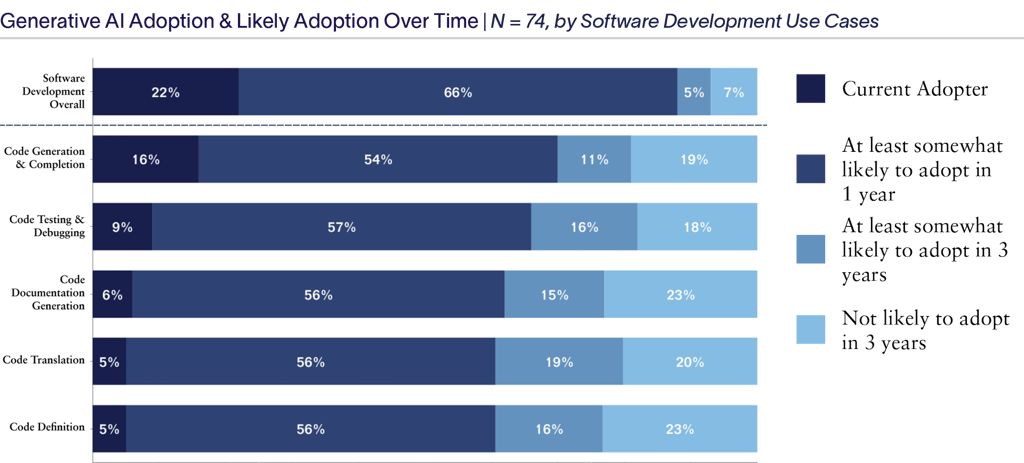
For marketers, generative AI is a valuable time saver in copywriting and image generation
Generative AI’s ability to craft words, images, and video is particularly valuable for marketers who develop and iterate collateral manually across different mediums and channels. Now, marketers can use tools like Jasper, copy.ai, or DALL-E to write blog posts, generate social media captions, provide SEO optimizations, and create custom art, all from a text prompt. 76% of marketers who have adopted or are planning to adopt generative AI noted its use for ”increased speed” on high volume, detail-oriented tasks like copywriting. While adoption is at 11%, future interest remains high: 74% of marketers believe they will be somewhat likely to adopt generative AI within the next year. Copy generation is the most common use of generative AI for marketing, with 9% current adoption, followed by image generation at 7% and video generation at 1%. Image and video generative AI models are less advanced than language models.
”If you’re a marketing strategist, you create content for TikTok, Instagram, Facebook, Snapchat, etc. Content for these platforms is all subtly different. With generative AI, you can create a lot more content easily, and you can create 100 different versions of the same thing.”
— Head of Product, Generative AI at a Model Provider —
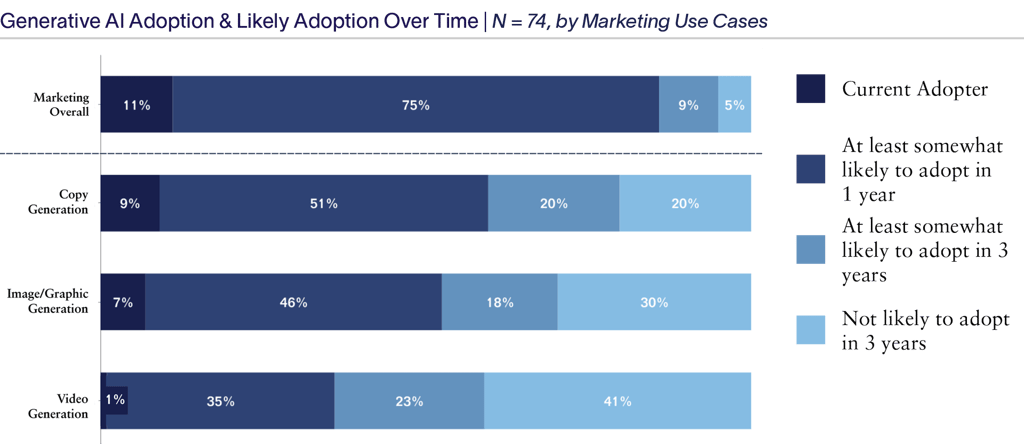
Customer service professionals deploy generative AI with caution
Customer service has long been heralded as an ideal business use case for generative AI. Industry executives agree that generative AI applications like Ada and Forethought provide a “human chattiness” upgrade from older, rule-based chatbots. However, according to our data, only 8% of customer service decision-makers currently use generative AI.
Existing customer service technology, such as virtual assistants like Amazon Lex and Azure Bot, is seen by industry experts as sufficient for the time being. Where software development and marketing functions can harness existing generative AI tools, enterprise chatbots require more customization, with special care given to boundaries and rule-setting. Customer service leaders are more risk averse when using emerging generative AI tools because the technology interacts directly with customers. Stories of misdirected generative AI chatbots providing incorrect or inappropriate responses pose a threat to customer service functions.
78% of customer service professionals not interested in adopting generative AI noted the technology had “unclear, insufficient value.” A Director of Product Management, whose enterprise has adopted generative AI, remarked, “Generative AI won’t outright replace old rule-based chatbots, at least not yet because I still don’t want to completely replace my doing-a-good-job current chatbot.”
Despite low current adoption relative to software and marketing, 71% of customer service leaders believe they will be somewhat likely to adopt generative AI tools in the next year.
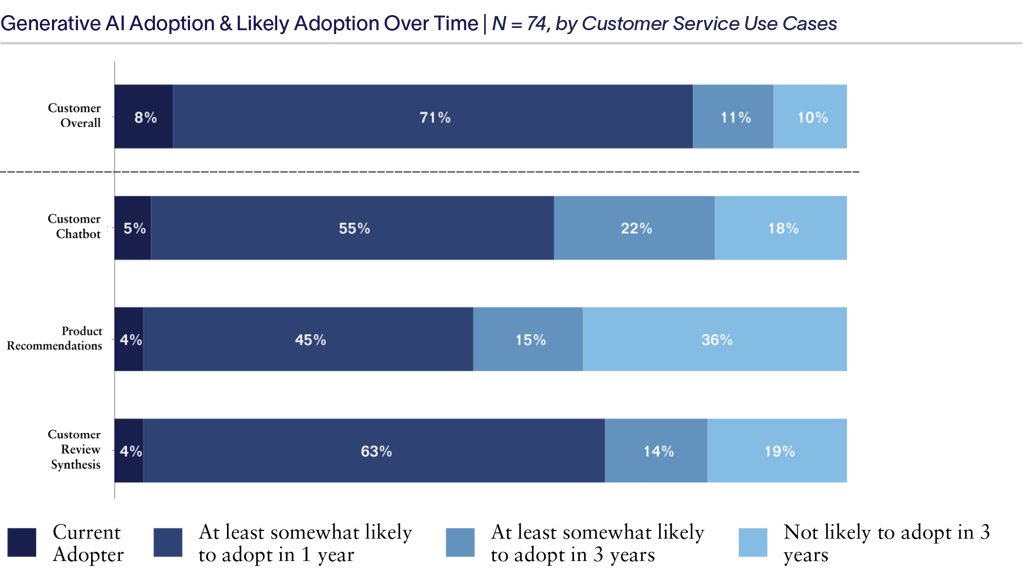
Product developers and product managers perceive generative AI tools as immature
Generative AI tools are slowly entering product development and product management. These include applications like nTopology, which design prototypes and renderings based on technical specifications, and tools that can create synthetic (but realistic) data for product design simulations. The adoption of generative AI tools for product development and management is currently at 6%, the lowest among the four business functions studied. Although generative AI has the potential to advance the product iteration process (the practice of designing, testing, and refining a product) for physical products, the underlying image-generating technology needs to mature to provide immediate value. 58% of current non-adopters of product management tools state that “tools are not established enough” for widespread use. We can imagine a future where the wide field of product development and product management will be assisted by various generative AI-powered solutions to speed up the design and iteration process.
”With regards to image models, the main use case is in design– specifically, new product ideation. Think of toy companies or product companies. You can quickly find social trends and create content to meet that demand.”
— Principal, Sales and Product Management at a Model Provider —
56% of organizations surveyed indicated they are somewhat likely to adopt generative AI technology for product development and management in the next year. Even though usage is low, the potential for generative AI to save time in product design has been noted. According to a Product Developer at an enterprise adopting generative AI, ”At a high level, 10% to 15% of product development work could be generated with AI. Though the percentage of people using it is small now, the impact of using the AI tool in terms of time consumption is great already. It has a proper impact even now.”
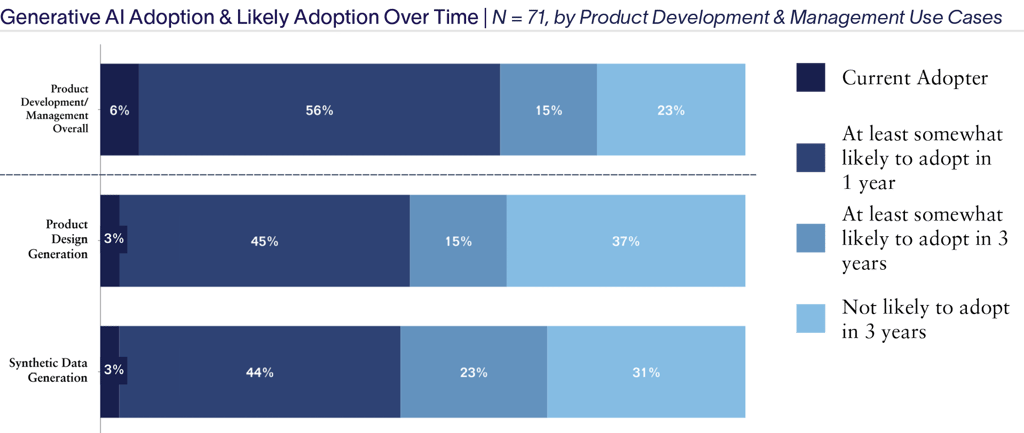
Generative AI is poised to transform key business functions, and companies need to consider development & deployment options & how adoption impacts compute and networking infrastructure
Generative AI can create content at a speed and scale that humans cannot match. Although the technology is still in its early days, professions as diverse as software engineering, marketing, customer service, product management, and product development are adopting these tools. And while generative AI tools can increase productivity and spur creative ideation, questions linger over accuracy, copyright infringement, data security issues, and regulation. Even leaders within the AI industry have voiced concerns about the rapid release of these products and their potentially negative societal effects.
As generative AI makes inroads into the enterprise world, businesses that integrate these tools need to be thoughtful in deploying and developing these solutions. The underlying technology will have to improve for generative AI to live up to its hype. Companies, infrastructure providers, and service providers must build an ecosystem to support this technology. We’ll go into further detail on how enterprises choose to deploy and develop generative AI and its impacts on underlying compute, storage, and networking infrastructure in the next installment of ”Putting Generative AI to Work.”
About the Analysis
In March 2023, Altman Solon analyzed emerging enterprise use cases by examining generative AI companies and capabilities. Utilizing expertise from a panel of industry executives, Altman Solon further investigated business activities with the most potential for immediate adoption and the highest momentum in the market: software development, marketing, customer service, and product management and design.
On these four functions, Altman Solon surveyed 292 senior executives and interviewed a panel of industry experts to understand opportunities for generative AI in businesses better. The surveyed decision-makers’ roles covered a range of strategic and technical functions, and the findings highlight adoption and adoption reasons for generative AI. All survey respondents were from the United States of varying business sizes.
This article was co-written with GPT-4. GPT-4 assisted in brainstorming and simplifying technical terms and concepts.
Complete the form below to receive a PDF of the full Generative AI report and to get first access to our next releases of "Putting Generative AI to Work."
Thank you to Elisabeth Sum, Oussama Fadil, Vivian Arsanious, and Joey Zhuang for their contributions to this report.